内容目录
文章来源于互联网:扩散模型=流匹配?谷歌DeepMind博客深度详解这种惊人的等价性
扩散模型和流匹配实际上是同一个概念的两种不同表达方式吗?
从表面上看,这两种方法似乎各有侧重:扩散模型专注于通过迭代的方式逐步去除噪声,将数据还原成清晰的样本。
而流匹配则侧重于构建可逆变换系统,目标是学习如何将简单的基础分布精确地映射到真实数据分布。
因为流匹配的公式很简单,并且生成样本的路径很直接,最近越来越受研究者们的欢迎,于是很多人都在问:
「到底是扩散模型好呢?还是流匹配好?」
现在,这个困扰已得到解答。Google DeepMind 的研究团队发现,原来扩散模型和流匹配就像一枚硬币的两面,本质上是等价的 (尤其是在流匹配采用高斯分布作为基础分布时),只是不同的模型设定会导致不同的网络输出和采样方案。
这无疑是个好消息,意味着这两种框架下的方法可以灵活搭配,发挥组合技了。比如在训练完一个流匹配模型后,不必再局限于传统的确定性采样方法,完全可以引入随机采样策略。
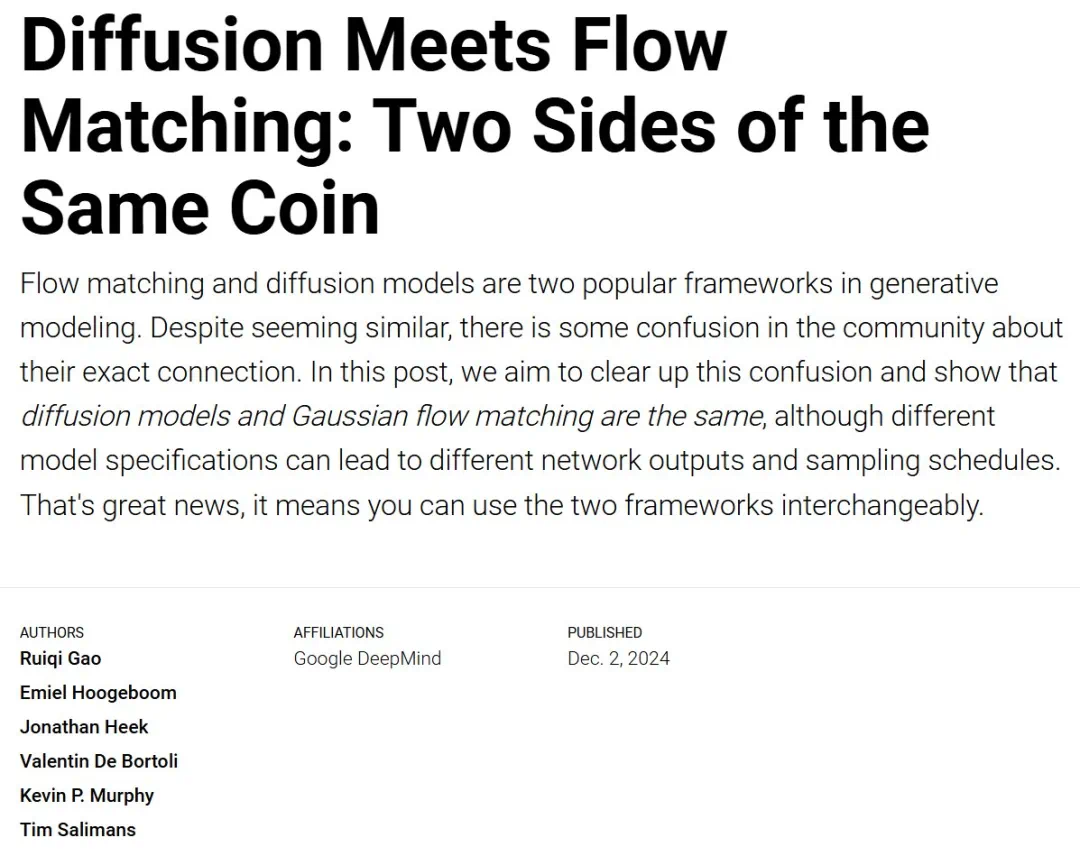

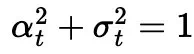








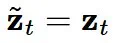




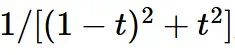
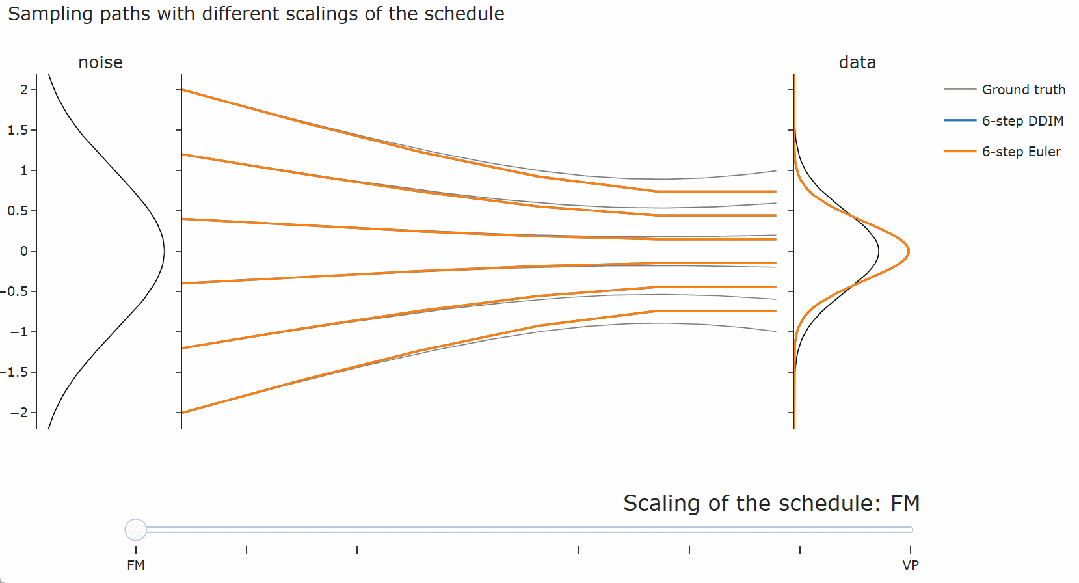
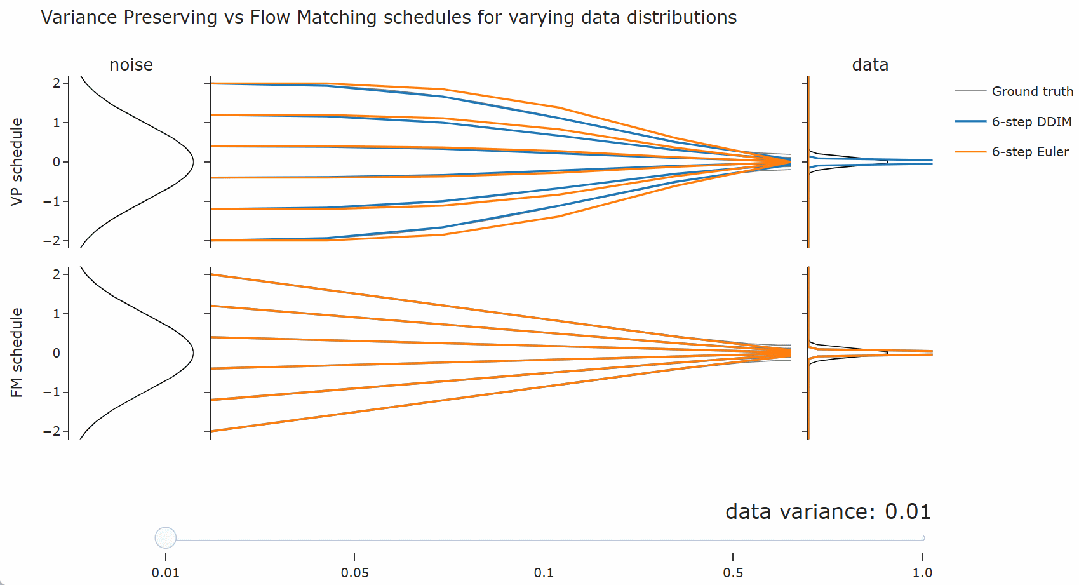
-
采样器的等价性:DDIM 与流匹配采样器等价,并且对噪声调度的线性缩放不变。 -
对直线性的误解:流匹配调度仅在模型预测单个点时才是直线。


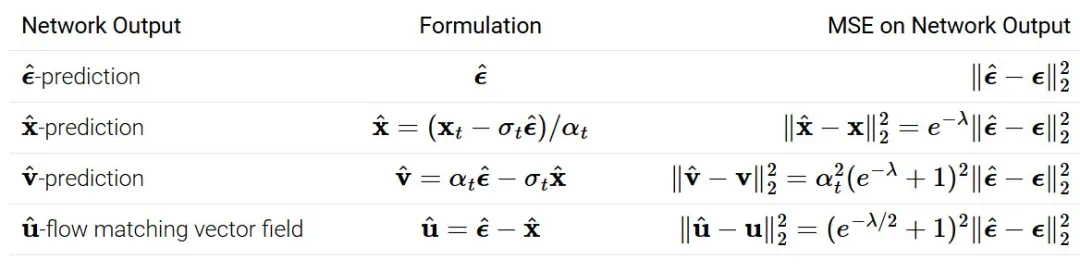
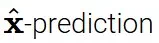



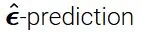
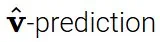


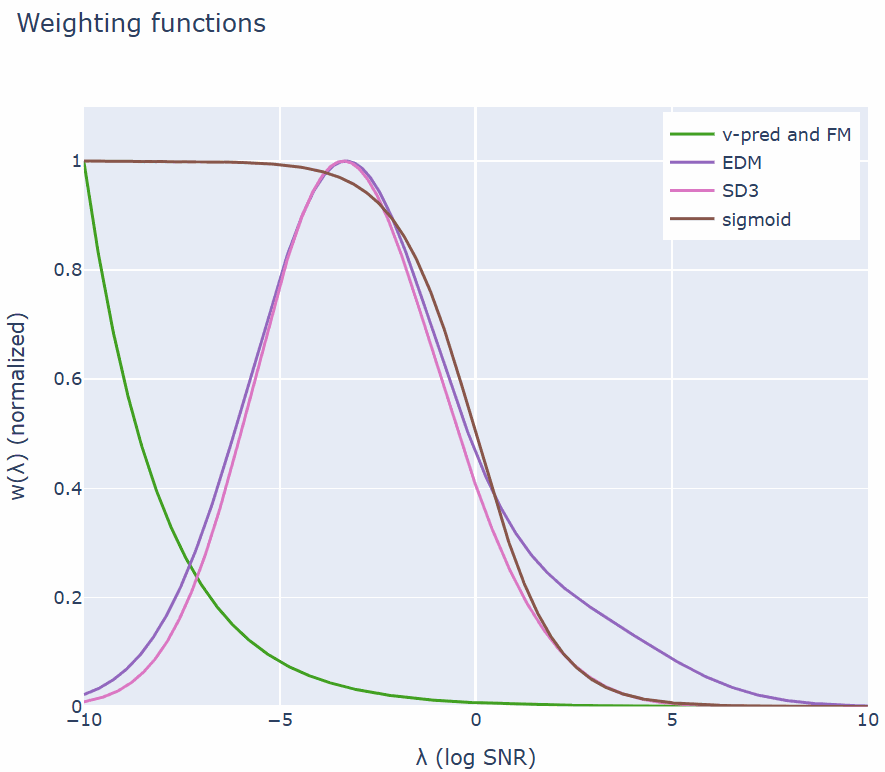

-
加权中的等价性:加权函数对于训练很重要,它平衡了感知数据不同频率分量的重要性。流匹配加权与常用的扩散训练加权方法相同。 -
训练噪声调度的不重要性:噪声调度对训练目标的重要性要小得多,但会影响训练效率。 -
网络输出的差异:流匹配提出的网络输出是新的,它很好地平衡了
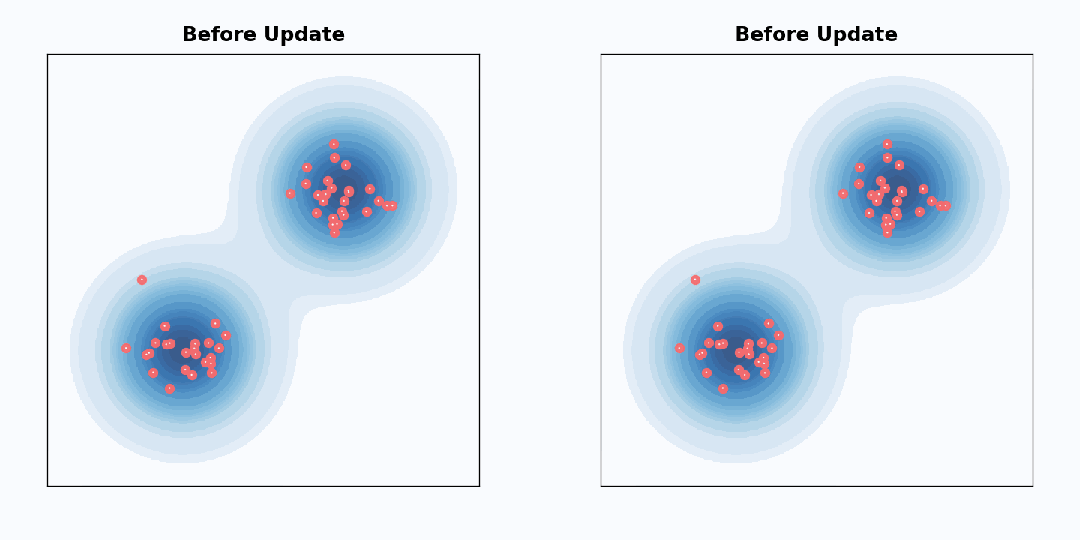
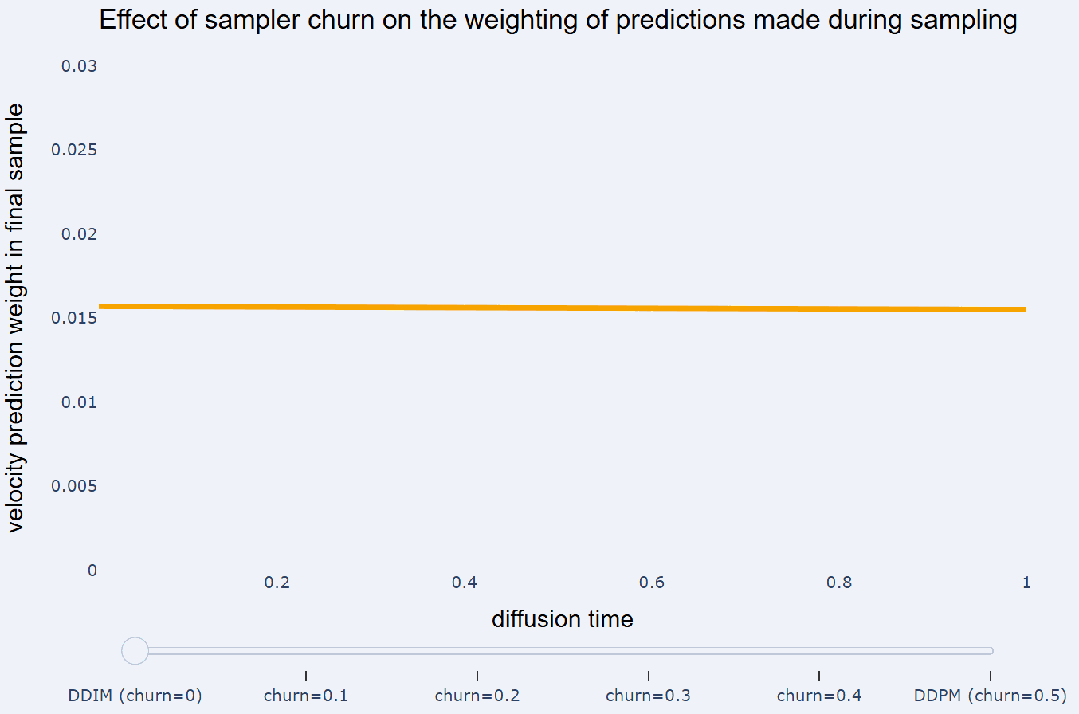



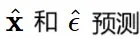







-
网络输出:流匹配提出了一种网络输出的向量场参数化方案,并且其不同于扩散文献中使用的方案。当使用高阶采样器时,网络输出可能会有所不同。它也可能影响训练动态。 -
采样噪声调度:流匹配利用了简单的采样噪声调度 α_t = 1-t 和 σ_t = t,并且更新规则与 DDIM 相同。
-
Flow matching for generative modeling -
Lipman, Y., Chen, R.T., Ben-Hamu, H., Nickel, M. and Le, M., 2022. arXiv preprint arXiv:2210.02747. -
Flow straight and fast: Learning to generate and transfer data with rectified flow -
Liu, X., Gong, C. and Liu, Q., 2022. arXiv preprint arXiv:2209.03003. -
Building normalizing flows with stochastic interpolants -
Albergo, M.S. and Vanden-Eijnden, E., 2022. arXiv preprint arXiv:2209.15571. -
Stochastic interpolants: A unifying framework for flows and diffusions -
Albergo, M.S., Boffi, N.M. and Vanden-Eijnden, E., 2023. arXiv preprint arXiv:2303.08797. -
Denoising diffusion implicit models -
Song, J., Meng, C. and Ermon, S., 2020. arXiv preprint arXiv:2010.02502. -
Score-based generative modeling through stochastic differential equations -
Song, Y., Sohl-Dickstein, J., Kingma, D.P., Kumar, A., Ermon, S. and Poole, B., 2020. arXiv preprint arXiv:2011.13456. -
Understanding diffusion objectives as the elbo with simple data augmentation -
Kingma, D. and Gao, R., 2024. Advances in Neural Information Processing Systems, Vol 36. -
Diffusion is spectral autoregression [HTML] -
Dieleman, S., 2024. -
Scaling rectified flow transformers for high-resolution image synthesis -
Esser, P., Kulal, S., Blattmann, A., Entezari, R., Muller, J., Saini, H., Levi, Y., Lorenz, D., Sauer, A., Boesel, F. and others,, 2024. Forty-first International Conference on Machine Learning. -
Elucidating the design space of diffusion-based generative models -
Karras, T., Aittala, M., Aila, T. and Laine, S., 2022. Advances in neural information processing systems, Vol 35, pp. 26565—26577. -
Knowledge distillation in iterative generative models for improved sampling speed [PDF] -
Luhman, E. and Luhman, T., 2021. arXiv preprint arXiv:2101.02388. -
Denoising diffusion probabilistic models -
Ho, J., Jain, A. and Abbeel, P., 2020. Advances in neural information processing systems, Vol 33, pp. 6840—6851. -
Progressive Distillation for Fast Sampling of Diffusion Models -
Salimans, T. and Ho, J., 2022. International Conference on Learning Representations. -
Dpm-solver++: Fast solver for guided sampling of diffusion probabilistic models -
Lu, C., Zhou, Y., Bao, F., Chen, J., Li, C. and Zhu, J., 2022. arXiv preprint arXiv:2211.01095.
文章来源于互联网:扩散模型=流匹配?谷歌DeepMind博客深度详解这种惊人的等价性